Heap Basic Concepts?
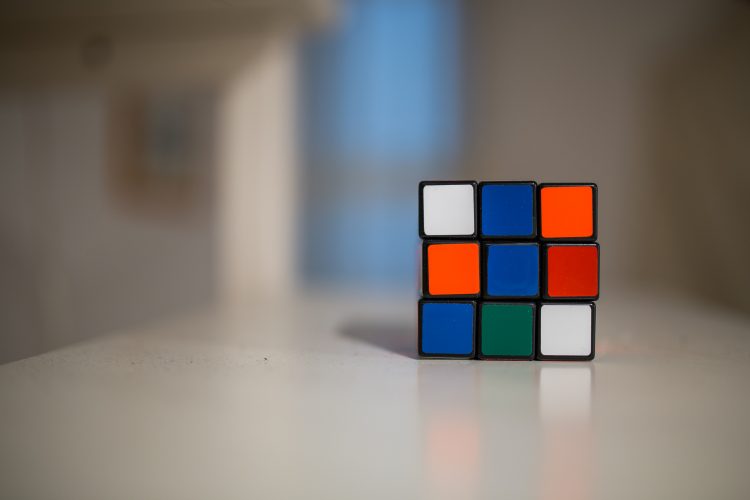
Heap Basic Concepts. Heap Operations. Question Examples Graph.
Heap (Priority Queue) is used to maintain the min/ max value within a dynamically changing data collection. 使用Heap来维护一个不断变化数据集中的最优值。 性质 -> 堆序性: 任意一个节点 小于 它的所有后续节点 descendent 逻辑 Logical 角度来讲:Theoretically, 在脑子里 Heap 是用 Complete Tree 来表示的。
为什么必须用 Complete Tree 来表示 Heap? According to 数学公式: index of leftChild = myIndex * 2 + 1 index of rightChild = myIndex * 2 + 2 index of parent = (myIndex - 1) / 2
为什么这个数学公式成立? 因为 Complete Tree 中间没有气泡
物理 physical 角度来讲: 在电脑里 Heap/ Binary Heap 是用 Array来进行存储的。
为什么可以使用 Array 来进行存储? Because it is a Complete Tree && We could easily get the parent & children index.
Heap Operations
Insert a new element: 对 Java 来讲是 offer(); Insert to tail (the first ‘null’ position).
Why? -> Need to ensure the tree is complete.
Swap with parent, if smaller than parent.
What is TC? -> Because it’s a complete tree, is must be a balanced tree, so O(logn).
delete heap-top element: 对 Java 来讲是 pop();
Swap the last not-null element with heap-top empty. Swap current element with min value among all its childrens. 这是唯一可以避免产生气泡的方式。要么补到 null 的位置,要么把前面的 null 换出来到结尾。 避免气泡是第一要素!!!
What is TC? -> Because it’s a complete tree, is must be a balanced tree, so O(logn). update any heap element:
if update to smaller: 往上 percolate/ swap ; if update to larger: 往下 percolate/ swap
get/ top: O(1)
Heapify: O(n)
Question Examples
Find smallest k elements from an unsorted array of size n.
Method 1:
Heapify the whole array to be a MIN_HEAP. -> O(n) Keep popping k times. -> O(klogn) Total TC: O(n) + O(klogn)
Method 2:
Heapify the first k elements to be a MAX_HEAP. -> O(k) Why heapify in this way? To keep a solution so far. Compare following elements with the Top-element, if smaller than the Top-element, pop the Top-element (踢出当前结果集) and insert the new element into Heap (收入当前结果集) -> O(logk) // otherwise ignore it. Total TC: O(k) + O((n-k)*log(k))
Method1 & Method2, which one is better?
k «« n: O(c*n) 这里有个系数c 由于Heapify产生的 VS O(nlog(k)) –> Hard to say.
k ~ n: O(nlogn) VS O(nlogn) –> Hard to say.
Method 3: 时间复杂度更优
Use Quick Selection:
Average Time Complexity: O(n)
Worse case:
iteration 1 -> O(n)
iteration 2 -> O(n/2)
iteration 3 -> O(n/4) …
Total Average Time -> O(n + n/2 + n/4 + … ) = O(2n) = O(n)
Method1 & Method2 & Method3 which one is most suitable for industry streaming data?
Method2 is an online algorithm, is does not depend on every data. No need to heapify every time, because we always keep the solution so far.
Graph
图的表示方法
Adjacency Matrix Adjacency List –可以优化成–> Hash Map
图中常考的 search algorithms
图的遍历和搜索 (BFS-1) 图中的最短路径问题 (BFS-2)
宽度优先搜索 BFS-1
什么是Search Algorithm? 以某种顺序, 把图中所有的 Nodes expand() 开, 并且 generate() 到它的所有 neighbors.
什么是 Breadth First Searcg Algorithm? 以宽度优先的顺序,把树(简易图)中所有的 Nodes expand() 开, 并且 generate() 到它的所有 neighbors.
面试中 如何去描述一个算法?
首先这个 algorithm 是干什么的? It is an searching algorithm which could expand every single Node and generate all its neighbors in a breadth first order.
其次 这个算法用的是什么数据结构? This algorithm uses the FIFO Queue.
这个算法是怎么 work 的? 其实状态是怎样? Initially, the Queue only contains the root node. 每一轮做了什么? We keep polling the head node from the queue, and expand it to generate its’ two child nodes. 终止的条件是什么? We terminate polling from the queue when it’s empty.